CONTENT
What is data science and why it matters for a business
Discover how data scientists analyze and transform data to extract knowledge, drive decisions, and gain competitive advantages.
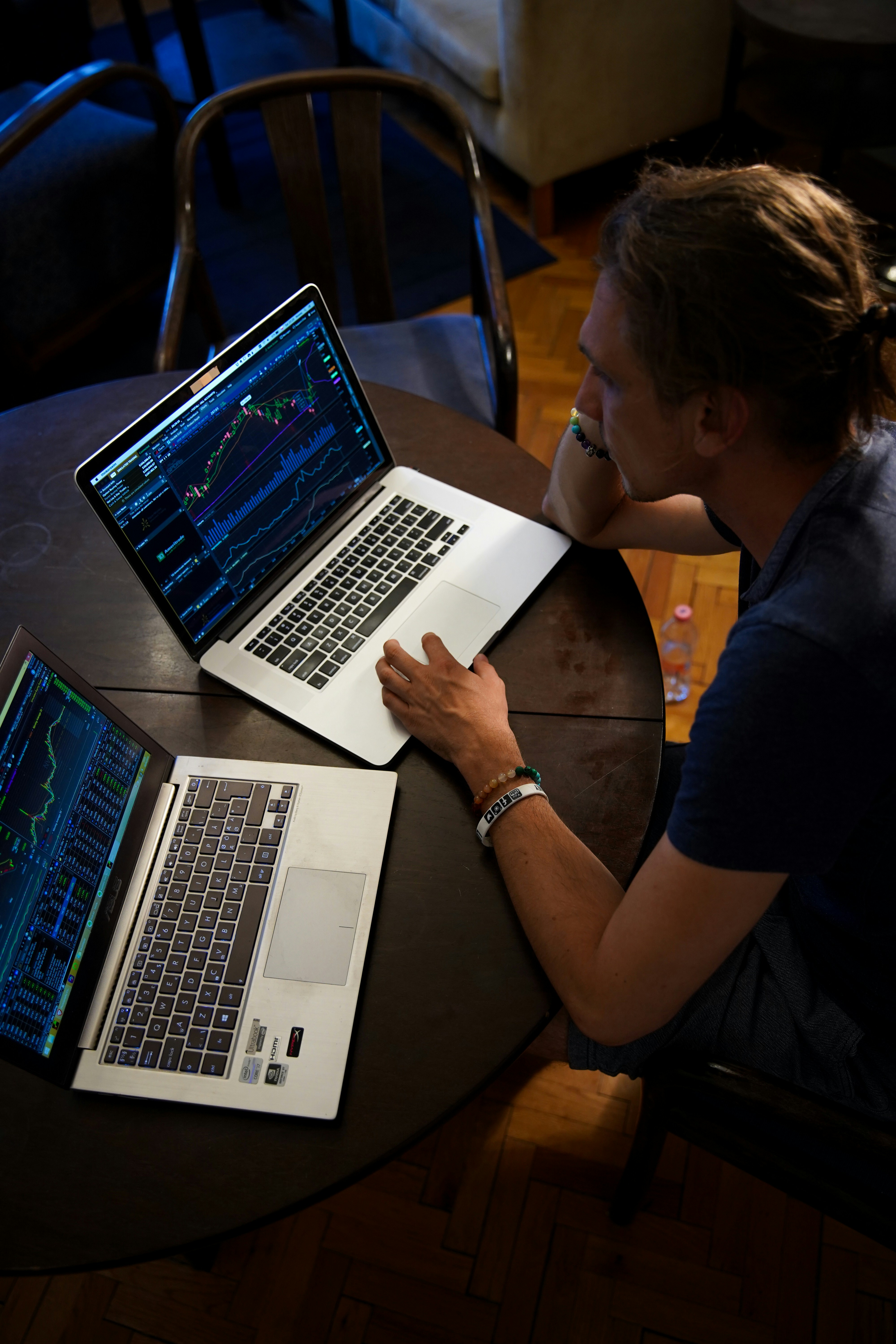
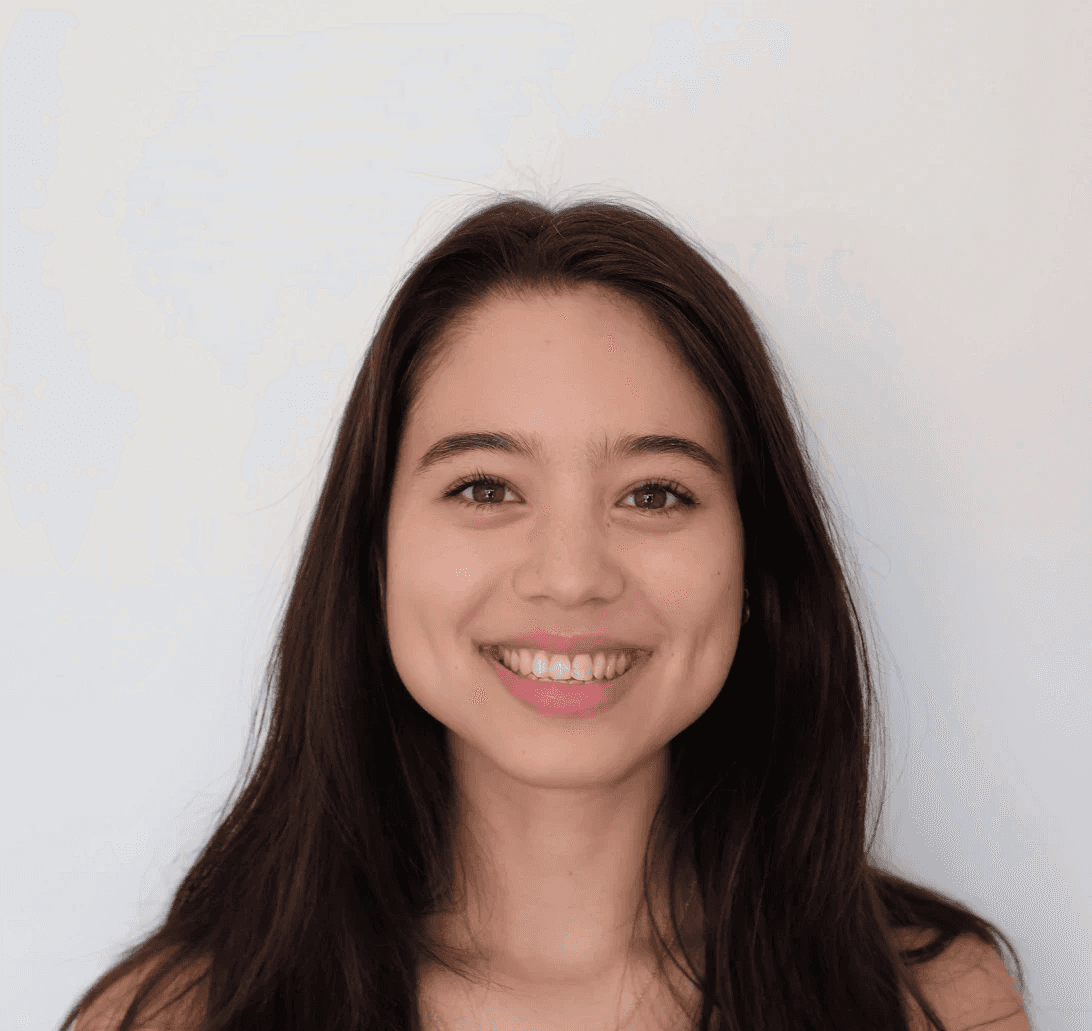
Saartje Ly
Data Engineering Intern
July 9, 2024
Data scientists collect, analyze, and transform data to extract knowledge and tell the underlying stories. Algorithms and methods are used on structured and unstructured data, combining elements from statistics, computer science, and domain-specific knowledge for data interpretation.
Key components of Data Science:
Data Collection: Gathering data from various sources such as databases, APIs, web scraping, sensors, etc.
Data Cleaning: Preparing the data by handling missing values, outliers, and inconsistencies.
Data Exploration and Visualization: Understanding the data through summary statistics and visual representations.
Statistical Analysis: Applying statistical methods to identify trends and relationships in the data.
Machine Learning: Building predictive models using algorithms that learn from data.
Data Interpretation: Drawing actionable insights and making data-driven decisions.
Applications of data science in business and marketing:
Customer Segmentation: Analyzing customer data to create distinct segments for targeted marketing.
Churn Prediction: Identifying customers who are likely to stop using a service or product.
Recommendation Systems: Personalizing product recommendations based on user behavior (e.g., Amazon, Netflix).
Sentiment Analysis: Analyzing customer reviews and social media to gauge public sentiment about products or brands.
The benefits of using data science include improved decision making, enhanced customer insights, and risk management. Data-driven insights lead to more informed and effective decisions, especially with understanding customer behaviours allowing for personalized marketing. Predictive analytics can forecast potential risks and enable the measures to mitigate them, and overall businesses find they have a competitive edge being agile and responsive to market changes.
Data scientists collect, analyze, and transform data to extract knowledge and tell the underlying stories. Algorithms and methods are used on structured and unstructured data, combining elements from statistics, computer science, and domain-specific knowledge for data interpretation.
Key components of Data Science:
Data Collection: Gathering data from various sources such as databases, APIs, web scraping, sensors, etc.
Data Cleaning: Preparing the data by handling missing values, outliers, and inconsistencies.
Data Exploration and Visualization: Understanding the data through summary statistics and visual representations.
Statistical Analysis: Applying statistical methods to identify trends and relationships in the data.
Machine Learning: Building predictive models using algorithms that learn from data.
Data Interpretation: Drawing actionable insights and making data-driven decisions.
Applications of data science in business and marketing:
Customer Segmentation: Analyzing customer data to create distinct segments for targeted marketing.
Churn Prediction: Identifying customers who are likely to stop using a service or product.
Recommendation Systems: Personalizing product recommendations based on user behavior (e.g., Amazon, Netflix).
Sentiment Analysis: Analyzing customer reviews and social media to gauge public sentiment about products or brands.
The benefits of using data science include improved decision making, enhanced customer insights, and risk management. Data-driven insights lead to more informed and effective decisions, especially with understanding customer behaviours allowing for personalized marketing. Predictive analytics can forecast potential risks and enable the measures to mitigate them, and overall businesses find they have a competitive edge being agile and responsive to market changes.
Data scientists collect, analyze, and transform data to extract knowledge and tell the underlying stories. Algorithms and methods are used on structured and unstructured data, combining elements from statistics, computer science, and domain-specific knowledge for data interpretation.
Key components of Data Science:
Data Collection: Gathering data from various sources such as databases, APIs, web scraping, sensors, etc.
Data Cleaning: Preparing the data by handling missing values, outliers, and inconsistencies.
Data Exploration and Visualization: Understanding the data through summary statistics and visual representations.
Statistical Analysis: Applying statistical methods to identify trends and relationships in the data.
Machine Learning: Building predictive models using algorithms that learn from data.
Data Interpretation: Drawing actionable insights and making data-driven decisions.
Applications of data science in business and marketing:
Customer Segmentation: Analyzing customer data to create distinct segments for targeted marketing.
Churn Prediction: Identifying customers who are likely to stop using a service or product.
Recommendation Systems: Personalizing product recommendations based on user behavior (e.g., Amazon, Netflix).
Sentiment Analysis: Analyzing customer reviews and social media to gauge public sentiment about products or brands.
The benefits of using data science include improved decision making, enhanced customer insights, and risk management. Data-driven insights lead to more informed and effective decisions, especially with understanding customer behaviours allowing for personalized marketing. Predictive analytics can forecast potential risks and enable the measures to mitigate them, and overall businesses find they have a competitive edge being agile and responsive to market changes.
CONTENT
SHARE